One of the application areas for agricultural IoT involves placing devices in the soil to detect moisture and using this information to drive more effective use of water. The primary goals are lower costs, higher yields, and environmental responsibility. These solutions have a lot of promise, but it’s essential to understand the potential complexities of implementation.
Device vendors combine sensors with a probe to reach the soil below ground level to take readings. Simple probes push into the earth at a low depth, making them suitable only for specific plants or ones grown in shallow pots or trays. For fields of crops, larger probes may require boring holes for placement. Also, measurements must go deeper, with some devices sensing 3 – 4 feet below the surface, often taking readings at multiple depths to increase the usefulness of the data.
Particularly for larger implementations, ease of configuration can be an important consideration. Some products are easily activated and connected to begin collecting and transmitting data. Others may require a more complicated process; however, the application and environment sometimes drive the product choice more than the simplicity.
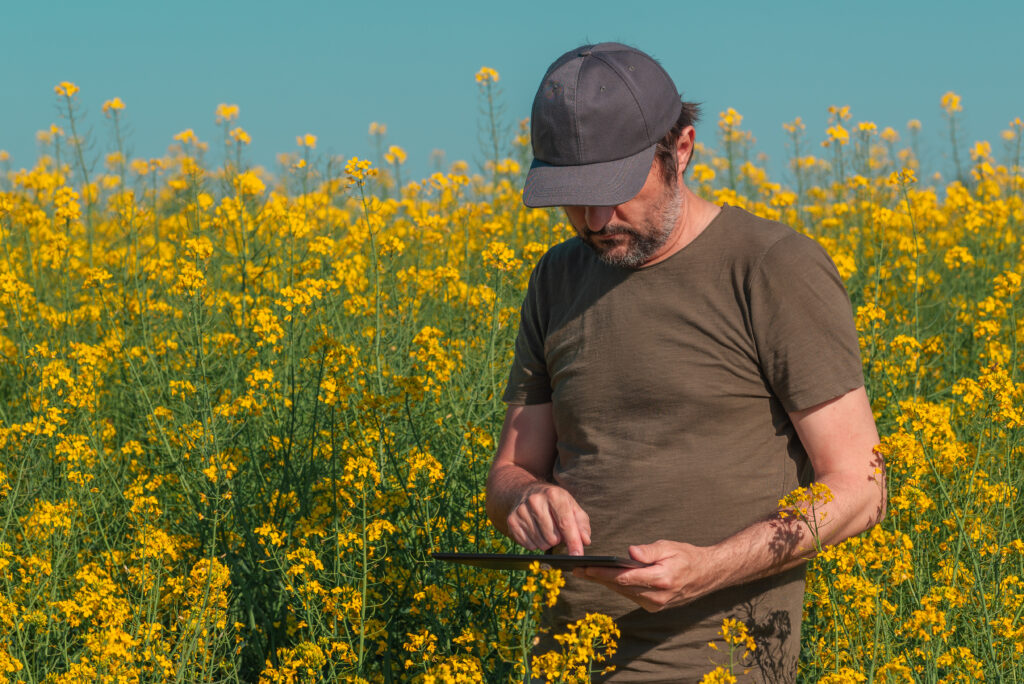
Assessing the moisture content of the soil provides information for crop health and input for irrigation decisions. Measuring the moisture level and the soil moisture “potential” can be helpful, requiring different sensors for each. Information about when to water and how much to apply is available when using both types.
Taking the additional steps to outfit irrigation equipment with sensors and remote controls for on/off, flow rate, etc., provides excellent complementary capabilities. “Smart Sprinklers,” for example, make watering measurable and precise.
Incorporating hyperlocal weather forecasts into the analysis can provide even more data for intelligent watering decisions. Installing connected weather stations local to the fields increases the accuracy of current conditions and can provide more precise weather predictions.
Connecting the probes to a network for data transmission is a must. The proper wireless technology selection is important when many sensors report data for large areas. Cellular connectivity may be a good choice for many implementations, but the typical recurring cost can make the solution expensive. In addition, coverage can be inconsistent (or nonexistent) in many areas where the human population is sparse and massive fields of crops are the norm. Here, a technology like LoRaWAN or mesh networking are good alternatives to consider.
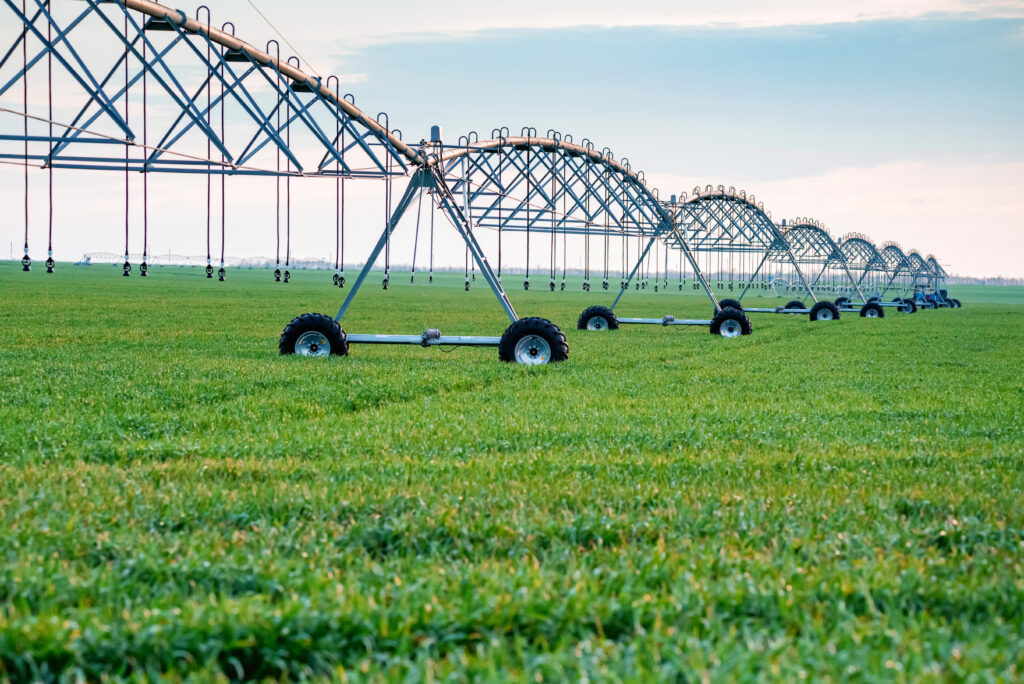
The next challenge is effectively using all available data once the implementation is complete. In addition to the information gathered by the sensors, crop-specific data about the optimum moisture conditions for maximizing quality and yield, characteristics of soil types, and other knowledge is important. Developing the best approach to ensure no under- or over-watering is usually best left to an expert agronomist.
Determining when to water requires monitoring and decision-making over time to get it right. The data may drive the implementation of a regular schedule, but making ad-hoc changes based on unusual conditions, such as significant temperature variations, refines the approach. When hyperlocal weather forecasting is a part of the solution, a delay in watering based on a high degree of probability for precipitation in the near term, for example, can produce better results, while also saving water that would otherwise be wasted.
Visualizing the data is vital, combining moisture, irrigation, and weather data. And overlaying the data on detailed maps augments decision-making and effectiveness.
The desired outcome is often a solution that requires little or no human intervention. In many cases, this is where Artificial Intelligence, specifically Machine Learning, can play an important role by improving the data’s usefulness, with intelligent automation being the ultimate goal.